A Primer on Good Judgment Inc and Superforecasters
At Good Judgment Inc (GJI), the official home of Superforecasting®, we pride ourselves on our ability to provide well-calibrated and insightful forecasts. As we continue to partner with clients and media worldwide, it is worthwhile to address some of the common questions we receive about our work. Here is a primer on our story, probabilistic forecasts, and our team of Superforecasters.
What’s in a Name? GJP, GJI, and GJ Open
The Good Judgment Project (GJP)
In 2011, the Intelligence Advanced Research Projects Activity (IARPA) launched a massive tournament to identify the most effective methods for forecasting geopolitical events. Four years, 500 questions, and over a million forecasts later, the Good Judgment Project (GJP), led by Philip Tetlock and Barbara Mellers at the University of Pennsylvania, emerged as the clear winner of the tournament. The research project concluded in 2015, but its legacy lives on. The GJP is credited with the discovery of Superforecasters, people who are exceptionally skilled at assigning realistic probabilities to possible outcomes even on topics outside their primary subject-matter training.
Good Judgment Inc (GJI)
GJI is the commercial successor to the GJP and the official home of Superforecasting® today. We leverage the lessons learned during the IARPA tournament and insights gained in our subsequent work with Phil Tetlock and his research colleagues as well as with leading companies, academic institutions, governments, and non-governmental organizations to provide the best and the latest in forecasting and training services. Our goal is to help organizations make better decisions by harnessing the power of accurate forecasts. GJI relies on a team of Superforecasters, as well as data and decision scientists, to provide forecasting and training to clients.
Good Judgment Open (GJ Open)
GJO, or GJ Open, is our public platform, open to anyone interested in making forecasts. Unlike GJI, which involves professional Superforecasters, GJO welcomes participation from the public. The “Open” in GJ Open not only signifies that it’s accessible to all but also draws a parallel to golf tournaments. Forecasting questions vary in their complexity, so there is no absolute score to indicate a “good” forecast. We use the median of participants’ scores as a benchmark, similar to par in golf, where lower scores indicate better performance.
A Note on Spelling
You may have noticed that “judgment” is spelled without an “e” on all our platforms. This is a consistent choice across GJP, GJI, and GJ Open, reflecting our preference for the parsimonious American spelling of the word.
Understanding Probabilistic Forecasts
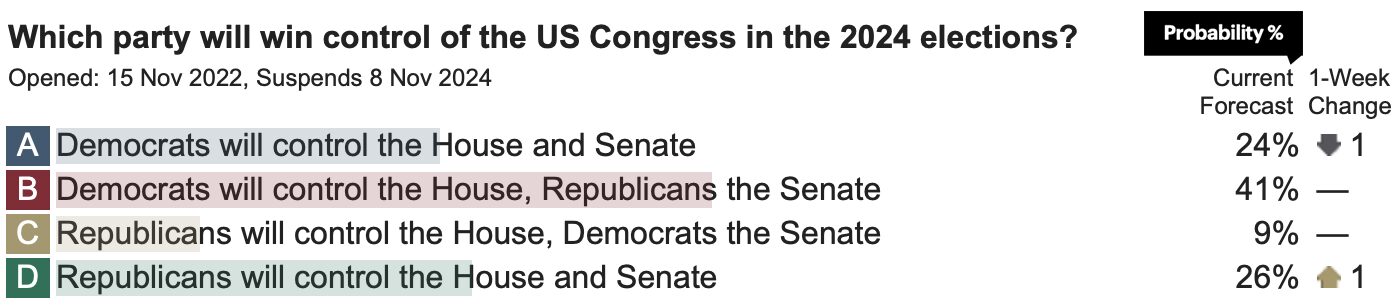
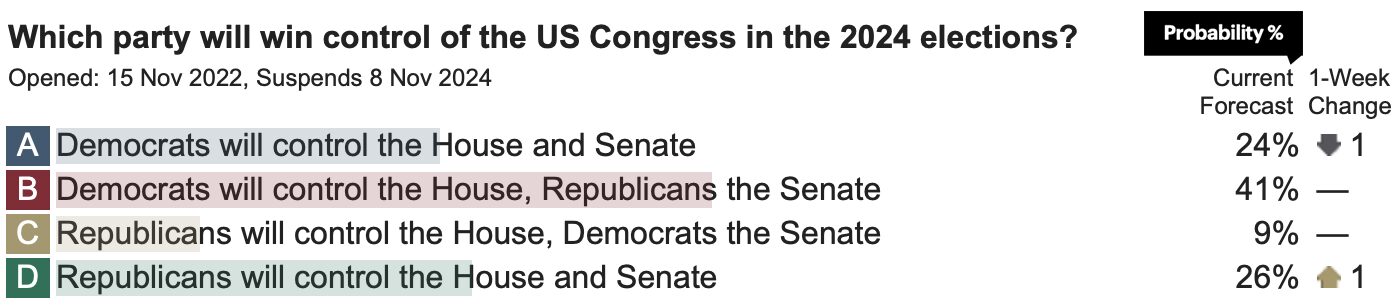
Our forecasts are not polls. They are aggregated probabilistic predictions about specific events. For instance, Superforecasters gave Joe Biden an 82% chance of winning the 2020 US presidential election. This means that if the election were held 100 times, Biden would win in 82 of those instances.
A common misconception is interpreting a probabilistic forecast as “X% of Superforecasters say a particular outcome will happen.” In reality, each Superforecaster provides their own probabilistic forecast, and we aggregate these individual predictions to reach a collective forecast. Therefore, an 82% forecast does not mean 82% of Superforecasters believe a certain outcome will occur. It is an aggregated probability of the outcome (an 82% probability of it occurring and an 18% probability of a different outcome) based on all individual forecasts.
Understanding Superforecasters’ Backgrounds
Age and Geographic Diversity
Superforecasters range in age from their 20s to their 70s and hail from different parts of the world. This geographic and demographic diversity helps to ensure that our forecasts are informed by a broad spectrum of experiences and viewpoints.
The Wisdom of the Crowd
We emphasize the importance of the wisdom of the crowd. Our Superforecasters read different publications in various languages and bring diverse perspectives to the table. To borrow terminology from Tetlock’s training materials in the GJP, some are Intuitive Scientists, others are Intuitive Historians, while still others are Intuitive Data Scientists.
Collaborative Nature of Forecasting
Forecasting at GJI is a team effort. We focus on collective intelligence. It’s not about individual forecasting superheroes tackling challenges alone but about identifying people who bring unique strengths to the table as a team of Superforecasters.
Get actionable early insights on top-of-mind questions by subscribing to our forecasting monitoring tool, FutureFirst™!